Have you ever heard about Machine learning (ML)? Probably yes, ML is a popular technique for network traffic classification and incident detection. However, have you ever heard about evaluating the quality of datasets (QoD)?
QoD is becoming more important with deployment ML in production, and project SAPPAN contributes to this topic.
The main prerequisite for any ML model is the input dataset that is used for training. If the input dataset contains mistakes and irrelevant data, the ML model cannot work correctly too. Moreover, the network traffic domain is a dynamic environment where patterns can change dynamically over time, and each network is also different. Therefore we need to take care of our datasets, measure their quality and improve them if necessary.
Currently, many authors publish public datasets but with limited descriptions. Therefore, we need to primarily trust the author’s reputation instead of verifying its quality for our network. The decision if we can use the available public dataset or create our own is repeated anytime we want to use the ML algorithm. This decision is not easy since there is no standardized way to evaluate the QoD.
Our recent study [1] is focused on initial definitions of dataset quality and proposes a novel framework to assess dataset quality. We introduce the following definitions that we are missing for standardized description of dataset quality: Good Dataset, Better Dataset, and Minimal Dataset. In Figure 1. you can see the high level architecture of our novel framework that we used for experiments to measure datasets quality.
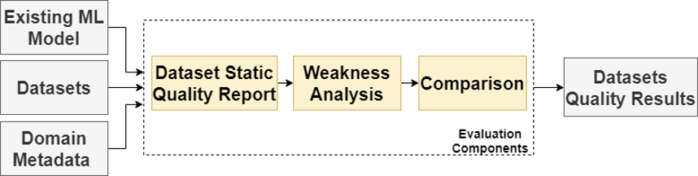
The aim of this framework is to find weaknesses and evaluate the quality of datasets with respect to the particular environment and domain. At the first stage, input datasets are statically tested to do an initial check of dataset format and Good dataset conditions. The second stage (Weakness analysis) is doing a dynamic evaluation of the dataset. Together with input metadata about the domain and ML model, we generate different datasets to see their bias. In the last stage, we compare different versions of generated datasets and optionally input datasets with each other to identify a better dataset. The output of the proces is a quality report with results, recommendations, and further optimization.
In the next steps, we would like to focus on the generation of datasets and the annotation pipeline that will automatically and continuously build our datasets and evaluate dataset quality. It is more important to generate and optimize a dataset for each target network than to leverage untrusted public datasets that do not have to match the patterns in our network. We are looking forward to pursuing the planned experiments and sharing our results.
References:
[1] D. Soukup, P. Tisovčík, K. Hynek, T. Čejka: “Towards Evaluating Quality of Datasets for Network Traffic Domain”, 17th International Conference on Network and Service Management (CNSM), 2021. To be published.